Care Coach
Combining Human-Centered Design and AI for Preventive Diabetes Care at Scale

Our journey working with SAP innovation center
Our team of five collaborated with the SAP Innovation Center Network, specifically targeting the healthcare sector. My responsibilities included research, stakeholder interviews, idea generation, wireframing, prototyping, and presenting our solution. We were honored to showcase our innovative solution at the SAP Innovation Center in Newport Beach, CA.

The evolution of our problem statement
Our client, SAP ICN, focuses on leveraging emerging technologies for healthcare enterprise solutions. They provided our team with an initial prompt: "How can we improve the healthcare shopping experience?" We initially explored broader healthcare issues before narrowing our focus, to prioritize diabetes management and scaling preventive care.
Why did we choose diabetes as our research focus?
Diabetes is a prevalent chronic disease in the U.S., projected to affect 54.9 million Americans by 2030. After our background research, we decided to focus on preventive care for type 2 diabetes. We discovered that it's preventable with a prediabetes diagnosis and lifestyle changes, offering significant impact.

The cost of managing diabetes is also growing rapidly. There is an opportunity for ICN and hospitals to scale preventive care to meet the projected growth in diabetes and reduce healthcare costs.
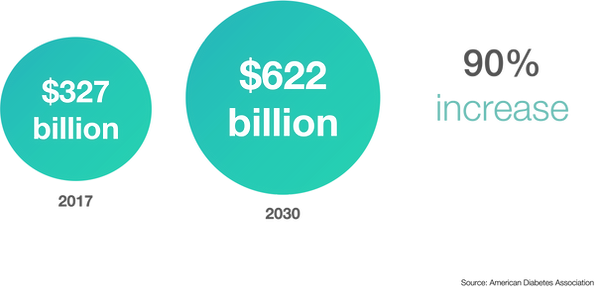
The problem
How might we design to predict and prevent type 2 diabetes at scale?
Challenges and insight from the hospital provider and patients
Our team faced significant challenges recruiting participants because we had a limited budget for compensation and limited time. We scaled our interview plan and used our networks to recruit. We spoke with 1 business analyst, 7 providers, and 4 patients. Our insights:

Diabetes demands close attention.
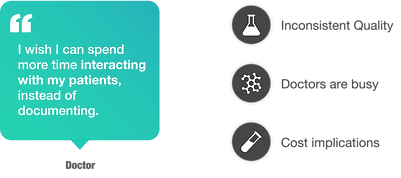
Caring for diabetes is also challenging for hospital
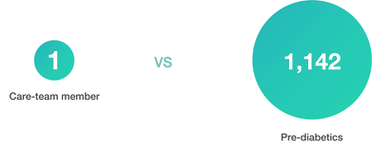
Hospitals need to scale care to meet demand
Prevention is the only cure
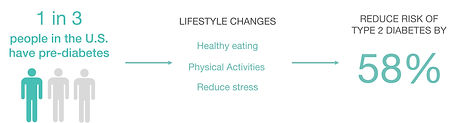
From research findings to potential solutions
During a week-long design sprint at SAP ICN's Newport Beach office, we shared our findings and received insightful feedback from the ICN team. While our initial insights centered on the patient experience, we recognized the need to pivot toward addressing the needs of healthcare enterprises, ICN's target market. Toward the end of the session, we brainstormed numerous solutions to tackle this challenge head-on.

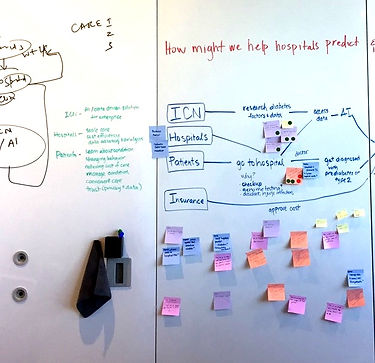
We explored several design methods, such as storyboarding, crazy eights, and experimenting with quick prototypes. Our brainstorming journey ranged from innovative wearables to AI care trucks. The the end, we focused on solutions that we believe are both visible and cost-effective for hospitals.
![]() | ![]() |
---|---|
![]() | ![]() |
Introducing our solution
Care Coach
This solution revolves around SAP's cloud-based analytics and AI platform. It can seamlessly integrate into hospitals through a unique private cloud partnership. The platform will offer recommendations based on the patient's risk assessment, drawing correlations from their clinical data, wearable data, and, when accessible, genetic data.
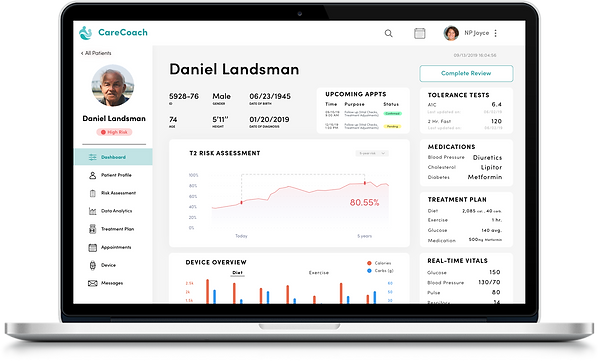
Diabetes risk assessment dashboard
Assess the patient’s risk for type 2 diabetes and recommend a preventive treatment plan.
Data-Driven Recommendations:
Clinical, wearable, lifestyle and genetic data collected about the prediabetes patient will power the AI.
Remote care
Tool to connect doctor and patients for distant care
Real time data
Providing real time data from patient wearable devices
How does Care coach do this?
Using the following data sets, SAP’s Leonardo will be able to calculate patients risk + care plan
Clinical
A1C
Fasting Glucose
BMi
Wearable
Heart Rate
Glucose
Body Temperature
Sleep Pattern
Lifestyle
Diete
Exercise
Stress Level
Generic
Genetics
Family History
Meet our persona
Nora

Take care of patients on daily basic
Sees value in leveraging technology to educate patient
Wants to get update of patient's treatment process
The Nurse
“Many diabetics are not ready to accept their condition. They think that eating fruits can help them!”
Dr. Chang
Very busy with their schedule
Overwhelmed with documentation
Wishes to spend more time with patients
Chasing time
“I wish I can spend more time interacting with my patients, instead of documenting.”

Daniel
62 years old
Diagnosed with prediabetes at age 46
Did not take physician’s advice to change diet and exercise more
Diagnosed with type 2 diabetes at age 51
In denial
“I wish I took my prediabetes more seriously.”

User flow and wireframe
We created two user flows for CareCoach. The first one outlines how a care team member can review a patient's health record and adjust the treatment plan for those at high-risk of type 2 diabetes.

Describe your image
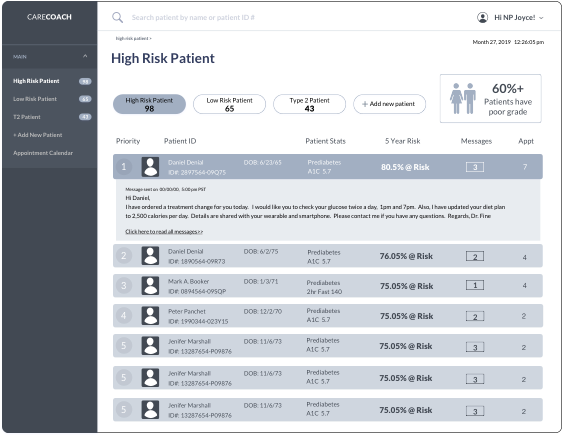
Describe your image
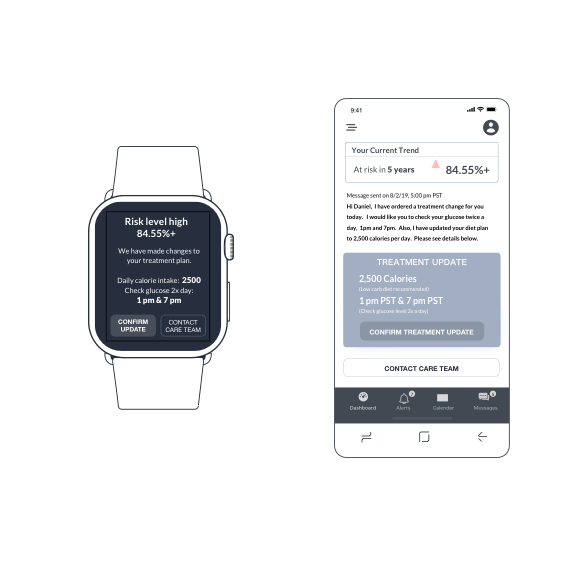
Describe your image

Describe your image
The second flow is for patients following their treatment plan and at low risk.
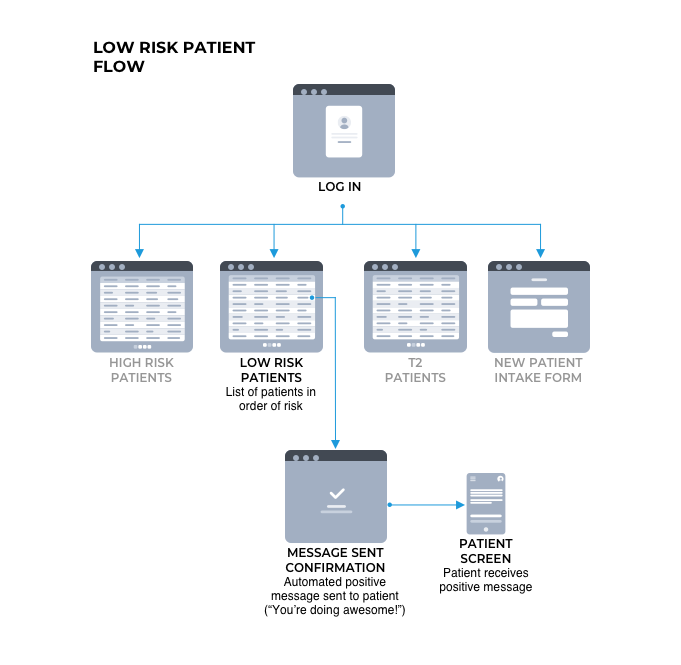
Describe your image

Describe your image

Describe your image
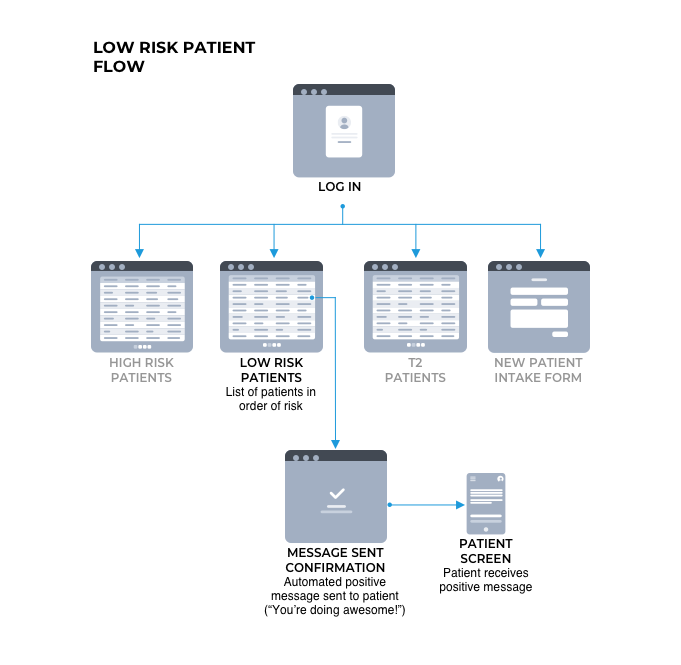
Describe your image
User testing
After conducting remote moderated tests with three doctors, we received valuable feedback for our prototype. We prioritized insights based on impact and severity, and realigned with the project scope to improve the wireframe. This led to the creation of the following high-fidelity design.
Key insights for improvement:
-
Transparency with AI risk assessment: Users seek clarity on how AI calculates patients' risk of type 2 diabetes and value visibility of collected data for system trust.
-
Integration with existing EHR system: Users desire clear access to existing EHR systems when using CareCoach.
-
Specific patient data feedback: Doctors emphasize data integrity, focusing on existing medical conditions, blood glucose levels, and carbohydrate intake.

Describe your image
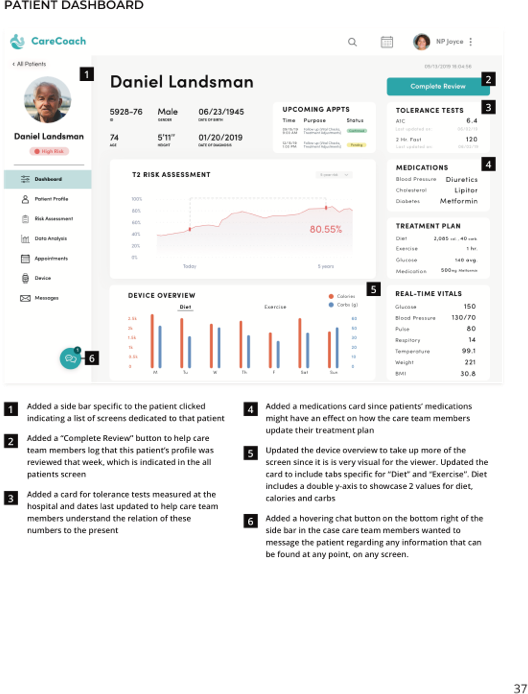
Describe your image

Describe your image

Describe your image
Presenting our solution
We presented our solution and conducted a prototype walkthrough at the SAP Innovation Center's product showcase event, attracting significant attention. Engaging in fruitful conversations, we observed genuine interest in addressing the identified problem.

The care team can see the list of all type 2 diabetes patients, with higher priority cases at the top.
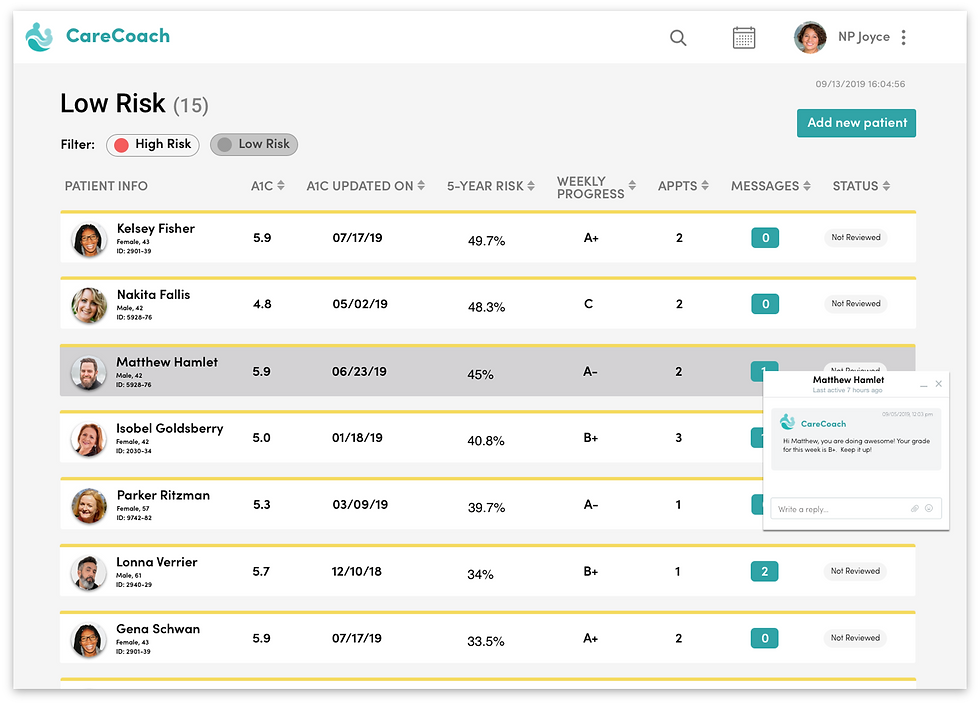
Low-risk patients who do not require intervention will still receive automatic messages from the system for reminders and encouragement.
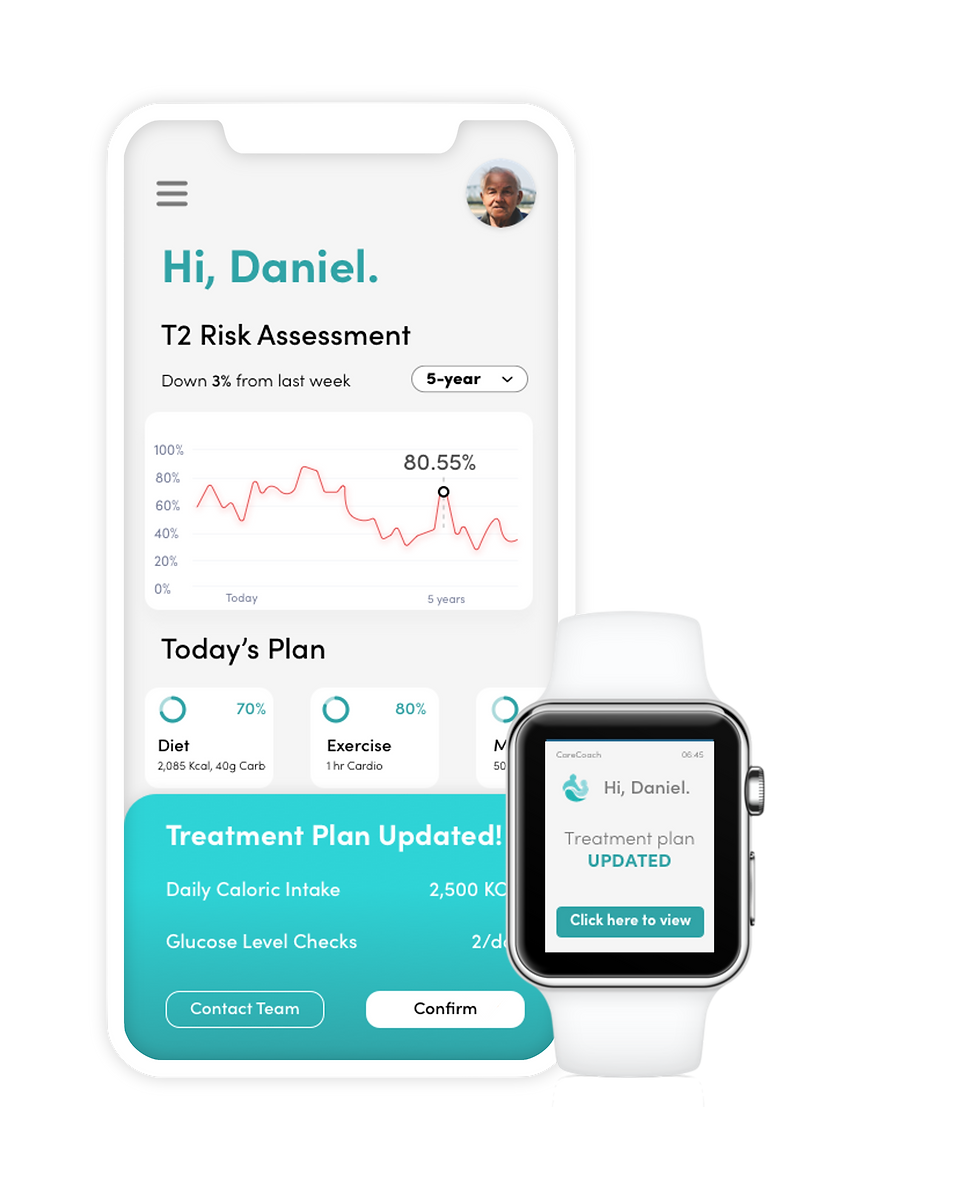
The patient's screen on their phone or wearable device allows them to connect with their doctor and helps the system collect data for diabetes risk assessment.

The care team can see the list of all type 2 diabetes patients, with higher priority cases at the top.
Next steps
-
Pilot Testing
Conducting small-scale pilot tests in real hospital environments to evaluate the effectiveness and feasibility of the AI system in predicting and preventing type 2 diabetes.
-
Regulatory Compliance
Ensure compliance with healthcare regulations like HIPAA to protect patient privacy and data security
-
Data Validation and Integration
Validate AI algorithms with real-world patient data and integrate the system with hospital databases and EHR systems for seamless data sharing.
-
Continuous Improvement
Establish mechanisms for ongoing monitoring, evaluation, and refinement based on user feedback and real-world data